Magic of Statistics: Your Ticket to Understanding the World
- Abhishumat Thakur
- May 5, 2024
- 4 min read
Updated: May 5, 2024
Do you ever wonder why numbers are like hidden keys to unlocking secrets? Well, that's where statistics comes in, like a spell-book revealing the mysteries of data.
But why should we bother learning it?
Let's take a fun journey to find out why statistics is important and what basic knowledge we need before diving into solving the Titanic dataset on Kaggle for hands-on learning .
Why Should You Read Ahead?
Picture you're on a grand adventure, exploring a wild jungle full of surprises.
Statistics is like your map, guiding you through the twists and turns of the jungle.
Understanding Everyday Life: Statistics helps you understand everyday things better, like why some days it rains and others it's sunny, or why some people like pizza more than burgers. From predicting the outcome of elections to understanding consumer behaviour, statistical insights shape our understanding of society.
Empowering Critical Thinking: In a world overflowing with information, statistics teaches us to critically evaluate data, distinguish between correlation and causation, and spot misleading conclusions.
Before we start our adventure, let's make sure we have all the tools we need
Here are some key concepts to grasp:
Data Types: Understanding the different types of data—such as numerical (quantitative) and categorical (qualitative)—lays the groundwork for meaningful analysis.
Numerical Data (Quantitative): Think of this like counting things. It's all about numbers! For example, if you're counting how many mangoes do you have, that's numerical data. It's stuff you can measure with numbers. But here's the interesting part, numerical data can be of two types:
Discrete Numerical Data: Now imagine you have a basket of mangoes. Now, let's say you want to count how many mangoes are in the basket. Each mango is like a little unit that you can count individually. So, if you count 1 mango, 2 mangoes, 3 mangoes, and so on, you're dealing with discrete numerical data. It's like counting marbles or toys—each item is a separate thing you can count, and you can't have "half" or "part" of a mango.
Continuous Numerical Data: Now, let's think about the weight of the mangoes. You can't really count the weight of each mango individually like you count whole mangoes. Instead, you can measure the weight using a scale, and you might find that one mango weighs 200 grams, another weighs 250 grams, and so on. The weight of a mango can be any value within a range, like 200 grams, 250.5 grams, or 307.8 grams. This is continuous numerical data because it's something you can measure, and it can take on any value within a certain range.
Categorical Data (Qualitative): This is more about describing things with words or categories. Imagine you have a bunch of different-types of mangoes. Say some are alphonso, some are kesar, and some are badami. That's categorical data. It's about putting things into groups or categories based on their qualities, like colour or type.
Measures of Central Tendency and Dispersion: Central tendency measures, including the mean, median, and mode, provide insights into the typical or central value of a dataset. They offer a snapshot of where the data tends to cluster.
Mean: This is like finding the average number of mangoes. If we count all the mangoes and divide by the number of different mangoes, we get the mean.
Median: Imagine lining up all the mangoes from the least to the most, and finding the mango right in the middle. That's the median. It helps us know if most mangoes are more or less than this middle mango.
Mode: This is the type of mango that appears the most. If there are more alphonso mangoes than any other colour, alphonso mango is the mode.
Standard Deviation (SD): This tells us how spread out the mangoes are. If the mangoes are all similar, the SD is small. If they are very different, the SD is large.
Variance: This is like a more detailed version of standard deviation, showing how much each mango differs from the mean.
Skewness and Kurtosis: These tell us if the distribution of mangoes is symmetrical or if it's stretched in one direction or another.
Probability Basics: Probability forms the backbone of inferential statistics, enabling us to quantify uncertainty and make probabilistic predictions. Understanding concepts like probability distributions, random variables, and probability rules is essential. We'll get to these in another post!
Embark on Your Statistical Journey
Armed with this foundational knowledge, you're ready to embark on your statistical journey. In the next chapters of our adventure, we'll explore descriptive statistics, where we uncover insights about datasets, and inferential statistics, where we make predictions and inferences about populations based on sample data.
So imagine we're setting sail on a grand adventure aboard the Titanic! But wait, this isn't just any voyage—it's a statistical journey through the Titanic dataset on Kaggle!
We'll use our newfound knowledge of statistics to explore the data and uncover insights about the passengers aboard this famous ship.
Get ready to don your captain's hat and embark on an exciting voyage filled with data, discovery, and maybe even a few surprises along the way. Are you ready to join us as we set sail through the Titanic dataset on Kaggle? Let's chart our course and dive into the world of statistics!
Thank you for going through this post, here have a mango!
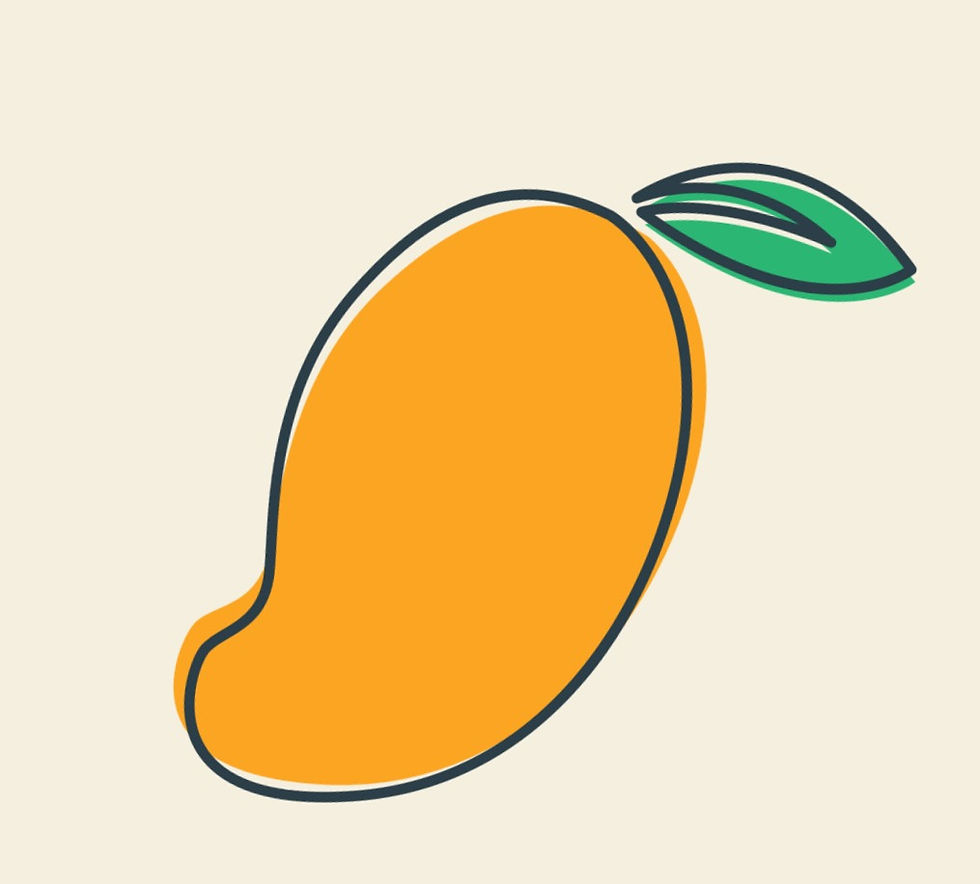
Comments